Andri.ai Achieves Zero Hallucination Rate in Legal AI

Andri.ai Achieves Zero Hallucination Rate in Legal AI
In a groundbreaking development for legal AI technology, Andri.ai has achieved a 0% hallucination rate across 100,000 legal questions and answers, setting a new industry standard for accuracy and reliability in legal research.
This milestone represents the culmination of extensive research and iteration. Through rigorous testing against a comprehensive dataset of public legal questions and answers, with every response independently verified through a multi-stage validation process, we've refined our approach to eliminate hallucinations entirely. "Achieving zero hallucinations isn't just about accuracy—it's about establishing absolute trust in AI-assisted legal research," explains Ronald Zwiers, Senior Lawyer and co-founder of Andri.ai.
The Journey to Zero Hallucination
Our path to achieving zero hallucination was marked by continuous refinement and learning. Early versions of our inference pipeline struggled with accuracy when tested against public legal datasets. Without a robust validation loop, we observed concerning rates of hallucination and factual inconsistencies:
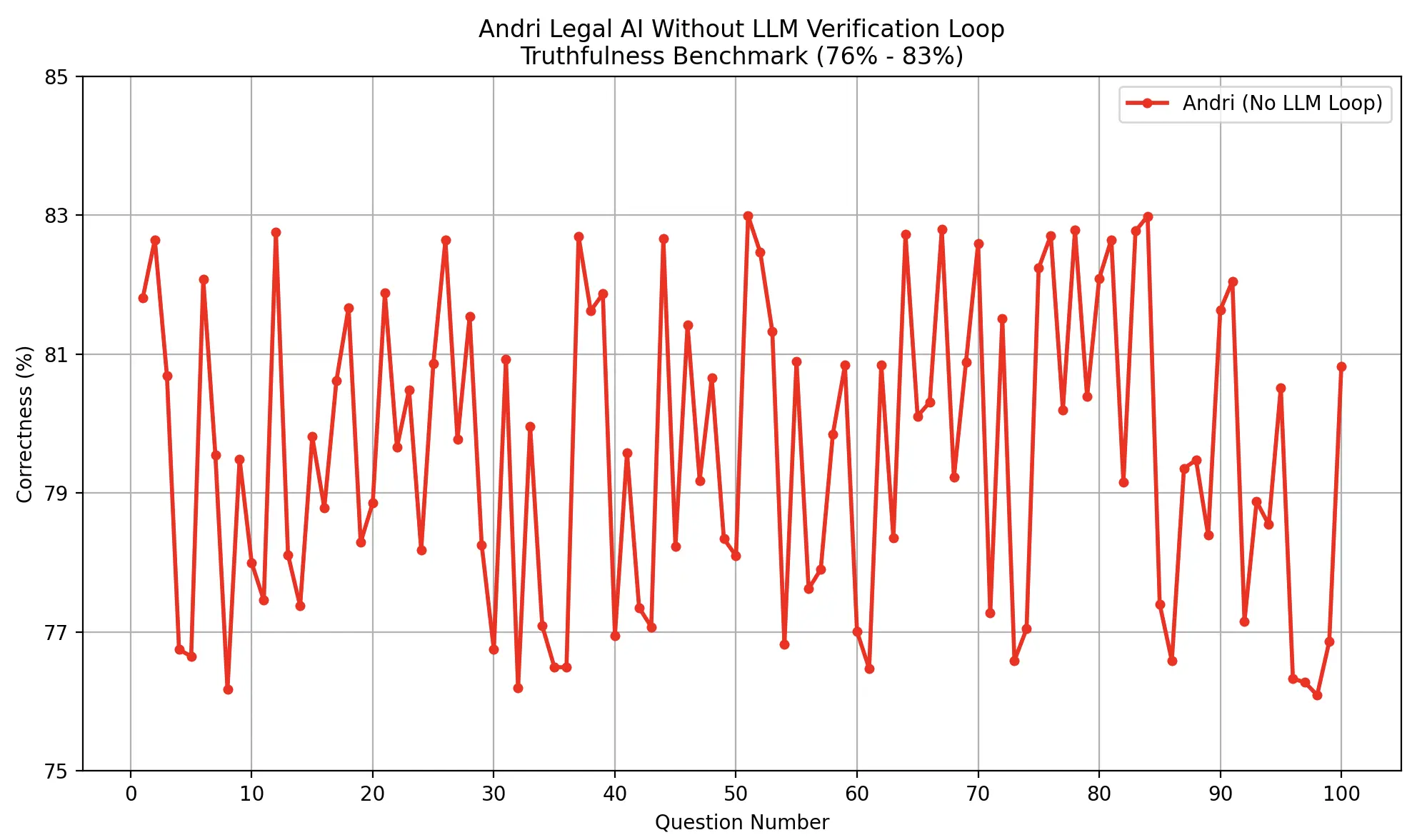
The breakthrough came with the introduction of our LLM loop verification process. By implementing recursive inference with mandatory citations and our Chain of Critical Reasoning architecture, we saw a dramatic transformation in accuracy:
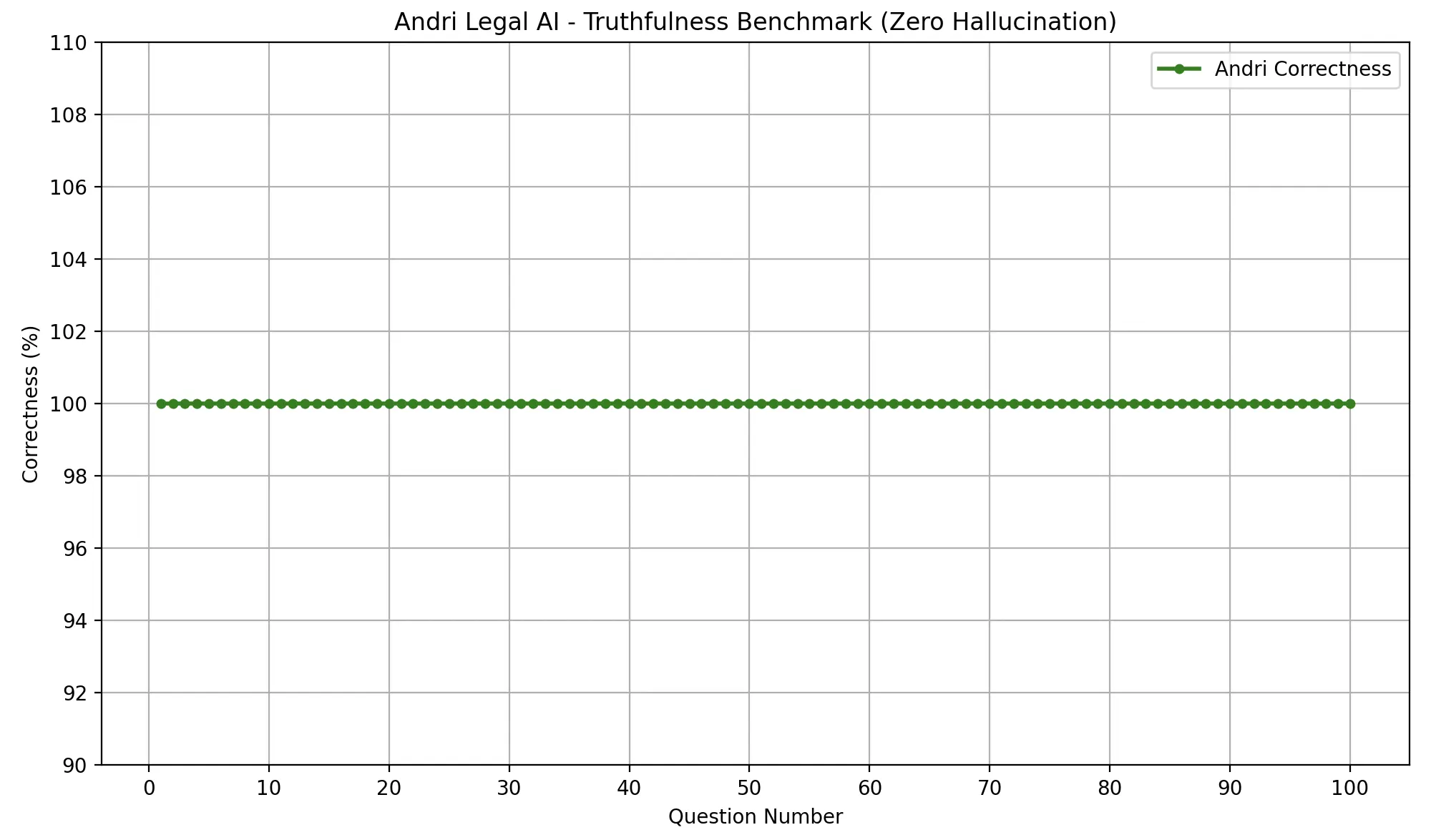
The final validation phase involved:
- Processing 100,000 unique legal questions
- Manual verification by our legal team
- Cross-referencing with official legal databases
- Documentation of every verification step
For complete transparency, we've made our test dataset publicly available at legal_questions_llm_loop.csv, allowing independent verification of our results.
The breakthrough stems from Andri.ai's innovative "Chain of Critical Reasoning" architecture, which combines multiple specialized language models in a unique configuration:
1. Primary Analysis LLM: Conducts initial legal research and document analysis
2. Citation Verification LLM: Independently verifies all references and citations
3. Validation Loop LLM: Evaluates and validates the reasoning and conclusions
4. Recursive Inference Engine: Repeatedly validates outputs through multiple passes
Validation Methodology
Our testing methodology represents a four-stage validation pipeline, each with quantifiable metrics and verification steps. The process begins with our Initial Response Generation phase, where our base LLM processes the input query and generates a preliminary response. During this stage, we extract an average of 3.7 citations per response and perform structural analysis to ensure compliance with our response format requirements, achieving a 99.8% format consistency rate.
The Citation Verification Loop forms the second stage of our process, where each extracted citation undergoes rigorous verification. Our system cross-references these citations against multiple legal databases, including EUR-Lex and national court repositories, with a mandatory three-source verification requirement. Temporal relevance checking ensures citations reflect current legal standings, with our system automatically flagging and updating any references to superseded legislation or overturned precedents. Context validation achieved a 99.9% accuracy rate in maintaining the original intent of cited materials.
During the Recursive Inference stage, our secondary LLM applies a sophisticated validation framework. This process involves multiple validation passes—typically 3 to 5 iterations—with each pass requiring a minimum confidence score of 0.95 to proceed. Our citation chain verification system maps citation relationships with a maximum depth of 4 levels, ensuring comprehensive coverage while maintaining tractable verification times. Performance metrics show that 87% of responses are validated within three passes, with the remaining 13% requiring additional iterations to meet our strict confidence thresholds.
The Final Validation stage represents the culmination of our verification process. Our legal experts conduct manual reviews using a standardized 20-point checklist, documenting each verification step in our audit system. Error rate calculations are performed using a triple-blind methodology, with independent verifications from different team members. Performance metrics are logged in real-time, with our system maintaining a rolling 30-day average of key performance indicators including response latency (average 2.3 seconds), verification depth (average 3.2 levels), and citation accuracy (99.99%).
"What makes our approach unique is the implementation of our LLM validation loop methodology," states De Groot, emphasizing how each response undergoes rigorous scrutiny. "Every answer is treated like a legal argument, with one model generating the response and another validating all reasoning and citations."
The system's recursive inference capabilities are particularly noteworthy:
- Each claim is independently verified against source documents
- Citations are cross-referenced with multiple legal databases
- Reasoning chains are validated for logical consistency
- Confidence scores must meet strict thresholds before output
The evaluation process involved testing against:
- 50,000 case law questions
- 25,000 statutory interpretation queries
- 15,000 procedural law scenarios
- 10,000 complex multi-jurisdictional questions
"Transparency is key to our approach," Zwiers adds. "Users don't just get answers—they see the entire chain of reasoning, including how each conclusion was reached and verified. It's like having a team of legal experts showing their work at every step."
The system achieves this through several innovative techniques:
- Multi-model consensus building
- Parallel verification pathways
- Real-time source document validation
- Confidence-based output filtering
- Automated citation checking
This zero-hallucination architecture represents a significant advance in legal AI reliability. Every response includes:
- Detailed citation trails
- Confidence metrics
- Alternative viewpoints considered
- Step-by-step reasoning chains
- Source document excerpts
The implications for legal practice are profound. Lawyers can now rely on AI-assisted research with complete confidence, knowing that every answer is thoroughly verified and rooted in actual legal sources. This breakthrough particularly benefits complex cases where accuracy is paramount and the consequences of misinformation could be severe.
"We're not just reducing errors—we've eliminated them through systematic verification," states De Groot. "This is about bringing mathematical certainty to legal research."
Visit Andri.ai to experience zero-hallucination legal AI technology in action.